Big tech says balance. But when AI treats lies and facts as equal, who loses?
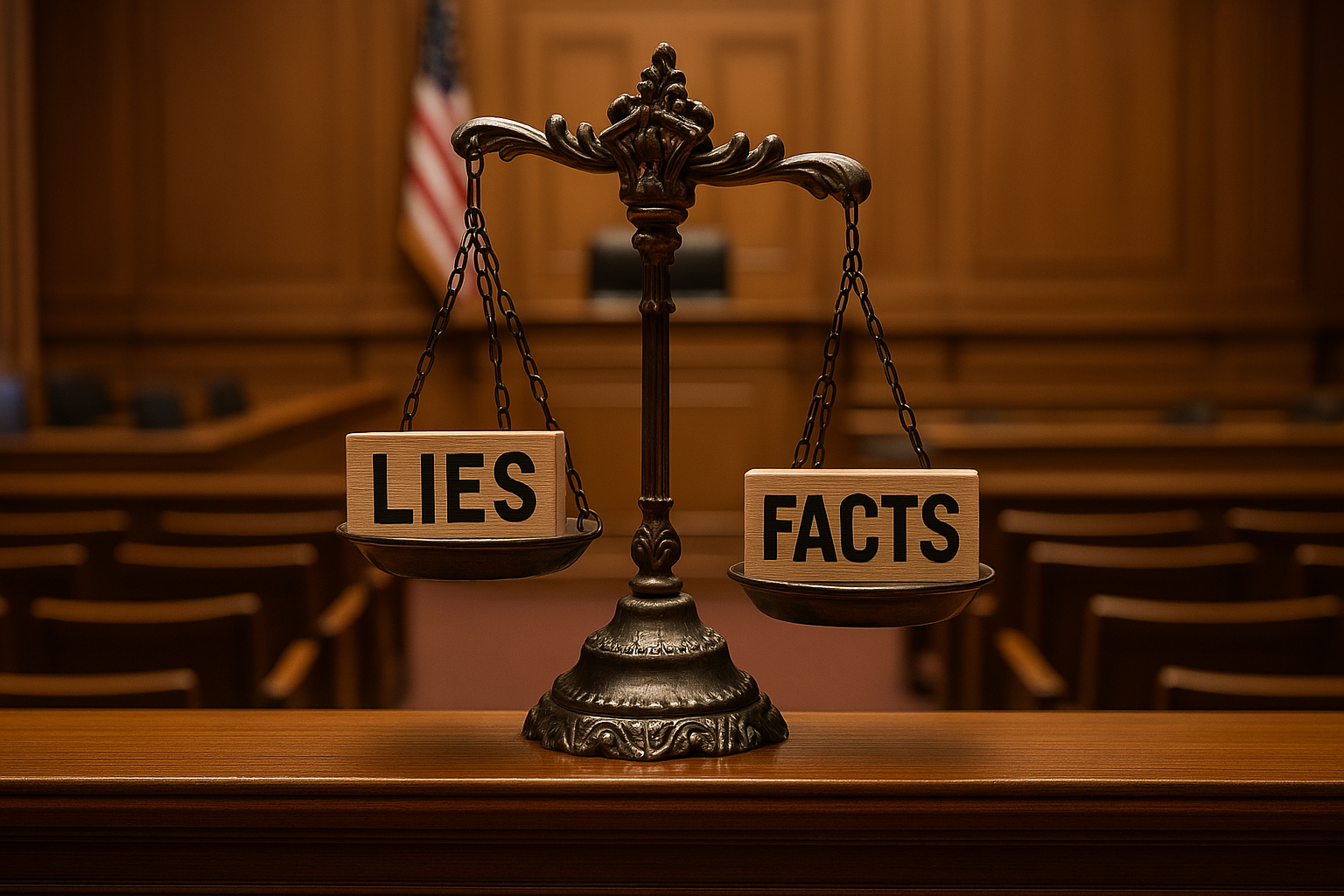
The Key Question
When Meta announced that its LLaMA 4 model would strive for “balance”—presenting “both sides” of controversial issues—I felt the familiar churn of frustration. The word sounds noble, even responsible. But in practice, this kind of framing often serves as a smokescreen, one that lets misinformation slip through under the banner of fairness. It was a Reddit thread reacting to Meta’s announcement that finally pushed me to write this piece. Because I keep seeing a troubling pattern: tech companies and media outlets invoking “bias” or “neutrality” to defend fundamentally flawed ideas.
This isn’t abstract for me. It’s not just ideology. It’s something I grapple with in real time as an educator and technologist. I’ve watched students—bright, curious, and diverse—engage firsthand with the limitations and risks of AI. And what they’ve uncovered makes one thing clear: “balance,” as we currently define it in tech, isn’t just insufficient—it’s dangerous.
The Classroom as a Test Lab
In a class of just ten students, I expected a range of attitudes toward AI. Some trusted it implicitly, others came in deeply skeptical. What surprised me was how quickly their assumptions—on both sides—began to unravel. Every assignment was designed to complicate things, to make them think harder, not feel smarter. That was the point.
In one module, we explored bias in image generation. The students noticed how AI consistently struggled to create Black characters in anime art styles. The lack of training data meant the model simply couldn’t improvise. In another assignment, students gave generative AI the same prompt rephrased in different styles—formal, casual, academic, poetic. The results? A stark reminder that the model defaulted to Euro-American storytelling conventions, even when the underlying meaning was unchanged.
But perhaps the most revealing moment came during a trial I ran while preparing material for future classes. I gave ChatGPT an official memo from the U.S. Department of Education. The memo, couched in formal and seemingly reasonable language, subtly suggested that DEI programs harmed white students. It was the kind of text that didn’t say the lie outright—but heavily implied it. And the AI swallowed it whole.
Not only did the model accept the memo’s claims uncritically, it treated the document as inherently valid—because it came stamped with the weight of officialdom. No cross-checking, no pushback. When asked to analyze it, the AI couldn’t read between the lines. At all. And that’s the problem.
When “Neutrality” Becomes Cowardice
These experiments reveal something that many people—especially those outside tech or academia—don’t realize: AI models aren’t just reflections of our knowledge; they’re reflections of our biases. And when a model is trained to treat all perspectives as equally valid, even ones rooted in bad faith or debunked claims, it doesn’t foster understanding. It creates confusion.
We’ve seen this dynamic play out in public discourse for years. We laugh off Flat Earthers, but anti-vaxxers still get prime-time coverage. In the name of “balance,” we legitimize viewpoints that actively undermine truth. There is an appropriate way to question science. But taking flawed studies out of context—or prioritizing feelings over facts—isn’t it. That kind of “balance” is performative, not principled.
In AI, the stakes are even higher. These systems are fast becoming de facto authorities. People don’t question the output of a chatbot the way they might question a journalist or a friend. If the model says it, it must be true. And yet we’ve proven, over and over, that these tools are often just repackaging misinformation with a confident tone and a shiny interface.
The Real Challenge: Teaching AI to Reason
I’m not anti-AI. In fact, I’m deeply AI-positive. I believe in its potential. But I’m also realistic about where it falls short. Today’s models can perform complex math and even excel in scientific reasoning. But when it comes to social reasoning—context, nuance, implication—they’re alarmingly weak.
They don’t know how to say, “That’s a lie dressed up in professional language.” They don’t know how to say, “This source is authoritative in appearance but vacuous in substance.” And until they can do that—until they can reason like humans in morally and socially meaningful ways—we’re going to have a serious problem.
This isn’t just a software issue. It’s a knowledge issue. A pedagogy issue. A public discourse issue. And it won’t be solved by aiming for artificial balance. What we need—what I’m trying to teach—is intellectual honesty. We need transparency, nuance, and models that can admit uncertainty rather than disguise it with faux neutrality.
Where Do We Go From Here?
My students aren’t discouraged by these discoveries. If anything, they’re energized. They see the flaws, yes—but they also see the possibilities. Every class session ends with more questions than answers, which is exactly how I want it. As an educator, I’m constantly hearing worries about the “dumbing down” of society thanks to AI. But I don’t subscribe to that view. People aren’t getting dumber—they’re facing tools that are too smart at sounding smart, without being right. The answer is not only teaching AI how to be better. The answer is also to re-invent education to meet the modern needs of instant AI statements, and the need for critical thought both in humans, and in AI.
So I say: let’s raise the bar. Let’s stop pretending balance is the highest good. Let’s teach AI—and ourselves—to ask better questions, to weigh ideas with care, and to push back against narratives that collapse complexity into false equivalence. If we don’t, we risk mistaking polished answers for real understanding.
Despite what some want to say, facts have no political bias.